Featured
How Machine Learning and AI Industry initiatives are shaping the oil and gas industry
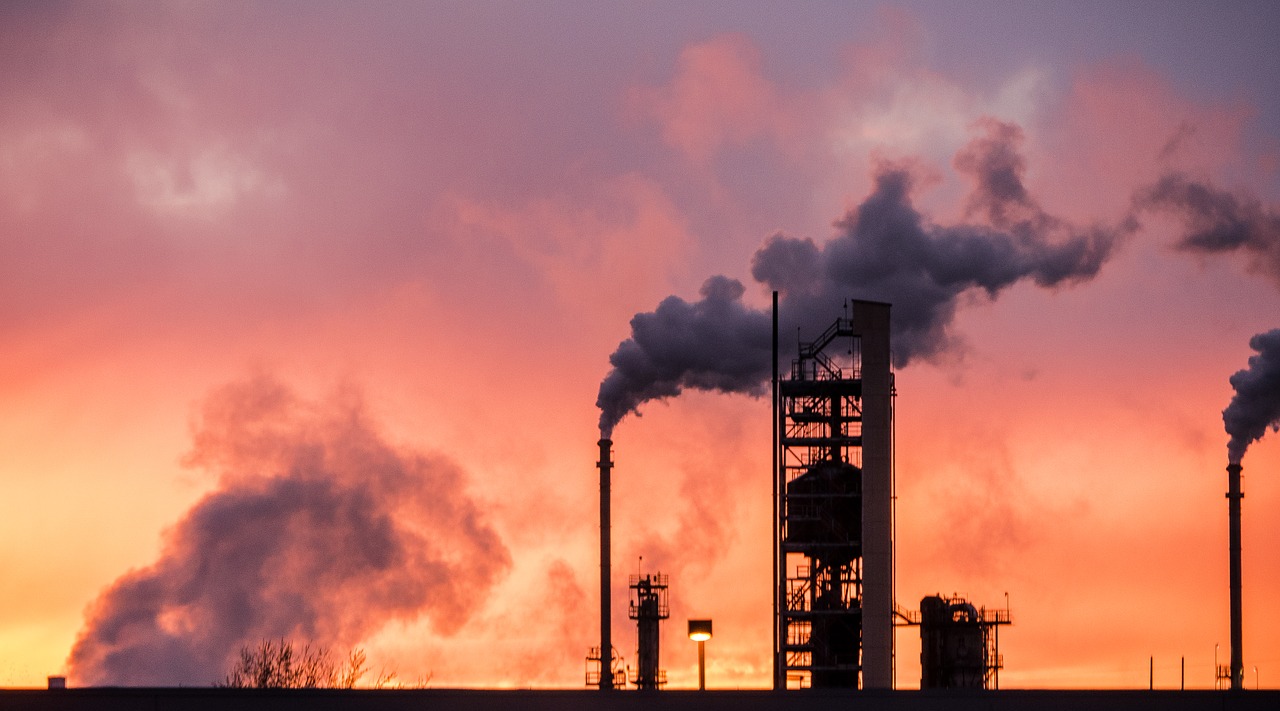

Vinodkumar Raghothamarao, Director Consulting, Energy Wide Perspectives & Strategy, IHS Markit EMEA
Oil and Gas companies operate in dynamic and complex environments, where they face constant challenges especially in terms of supply and demand. Oil and Gas companies have been adopting digital technologies for years, helping to increase the recovery of fossil resources, improve production processes, reduce costs and improve safety.
Oil and Gas companies are emerging from the downturn leaner and more efficient. Although technology and broader forms of innovation certainly deserve some of the credit for these gains—especially around enabling lower-cost design concepts, raising well productivities, and improving coordination among functional groups and assets—the call on technology is likely to rise substantially over the coming years.
As the oil and gas industry continues to adapt to a sustained period of volatility, companies are taking concrete steps to reduce costs and raise efficiencies. Technology and innovation are at the heart of many of these efforts and in certain “pockets of excellence” are helping to reduce facility costs by 5–15%, lower operating costs by 10–70%, and raise production efficiencies by 5–20%.
The oil and gas sector has a relatively long history with digital technologies, notably in upstream, and significant potential remains for digitalization to enhance operations further. Now with the oil prices steadily increasing, the time has come to evaluate, adapt and embrace new technological initiatives. Machine Learning and Artificial Intelligence are the two key technological initiatives driving the tectonic shift within the oil and gas industry.
Machine learning and other big data applications could save the oil and gas industry as much as $50 billion in the coming decade, according to McKinsey. Since the cratering of the global oil price in late 2014, companies have increasingly been looking at technology to reduce costs, improve efficiency and minimize downtime.
Machine learning, the ability for computers “to learn without being explicitly programmed” is one of the subfields of the big data revolution has been applied to modern business since the 1990s, and is coming to the aid of the hydrocarbons sector in the oil price slump.
Of all the parts of the oil and gas industry rife for the rollout of machine learning, the upstream sector is the obvious choice.
Reliant on Bayesian statistics, a branch of mathematics that employs ”degrees of belief” to interpretations of probability, machine learning creates and uses algorithms to make predictions on data. The exploration phase, dependent on the interpretation of layers of information riddled with uncertainties, is a perfect fit for the machine learning approach.
The rapid identification of patterns working across multiple variables had been a time-intensive process; this can now be partially-automated and optimised with oversight from experienced professionals. Reservoir modelling, and trying to find out how a formation will react to particular drilling techniques can also be verified using a combination of algorithms and fuzzy logic, a technique used for prediction when information is either unreliable and/or incomplete.
By the same author: Best Practices in Oil and Gas Supply Chain and Procurement
Testing the waters “virtually” before a drill ever disappears downhole could save a company anywhere between millions and billions. Looking back at past failures and applying the lessons learned is a fundamental problem solving technique.
Machine learning can speed up this process and widen the search net when seeking prior instances of similar problems in a case library. Trawling through a database of logged events for a description that matches the issue at hand, solutions can be accessed in real-time to provide an essential guide for personnel on the ground to use as a troubleshooting tool.
Deep learning (which is one of the method of Machine Learning) and the Internet of Things (IoT) are two aspects of artificial intelligence (AI) that could potentially revolutionise the oil and gas industries. Having already made quite a storm in various other industries including consumer electronics, this couldn’t come at a better time for the oil industry as it currently faces dramatic drops in the price of oil. While there are no doubt several AI practical applications already in place that will indeed help these industries improve the following are three have the potential to make a significant difference across the board.
1. Rig diagnostic bots: In the same way that bots are being used in customer service departments, field technicians can interact with diagnostic applications through voice controls. This is made possible through the use of deep learning and and natural language processing algorithms and enables remote diagnostics at the touch of a button.
2. Risk detecting deep learning algorithms: It’s very difficult for a human to be able to see in all the nooks and cranny’s of pipelines, so instead scientists and computer programmers use algorithms to reveal patterns and weaknesses that may have otherwise been left undetected. Drones are now being used at a number of oil and gas sites to inspect pipelines. They are able to record footage in real-time as they fly through the pipelines trying to detect any cracks or leaks. Once the drone footage has been received deep learning algorithms set to work finding any pixel signatures that may indicate a crack or leak.
3. Using deep learning algorithms to spot anomalies: Various oil and gas companies have benefited from using sensors attached to equipment such as rack rods or rod pumps in which to gather data. But, trying to detect anomalies in this way is very difficult. Using deep learning algorithms, experts can see anomalies that conventional methods would have missed and can alert the rig’s command centre in advance.
4. One key area of the industry that AI could help with is in sourcing the best drilling locations. Currently there are far too many false positives when it comes to locating suitable places to drill for oil, and AI could cut that number down significantly. AI could also be used to determine the most cost-effective shipping routes for vessels carrying gasoline while taking factors such as whether into consideration.
5. Other areas that AI will be used in the energy industry include robotic automation various tasks including ship vetting, and improved automated drilling.
By the same author: What does BlockChain hold for the Energy Industry?
Drilling process in upstream sector is one of the riskiest and expensive ventures, hence this needs to be accomplished with detailed planning and effective execution. Applying ML and AI in the operational planning and execution stages can help improve the well planning process significantly, real-time drilling optimization, friction drag estimation, and other well prediction. In addition to this, the technique can also be applied in the field of geophysical aspect, where the well variables like the Rate Of Penetration (ROP) improvement, well integrity, drilling equipment condition recognition, real-time drilling risk recognition, and of course the operational decision making.
A lot of factors need to be taken into consideration while applying techniques like machine-learning in drilling. Few to name are the traditional data such as pressure differentials at various points, thermal gradient, permeability, porosity, and seismic vibrations.
Reservoir Management – Reservoir being the core of the entire operation in upstream, needs to be maintained and optimized to increase the longevity of the whole production cycle. Therefore, reservoir, facility and the well need to be managed, and this requires an integration of multiple disciplines, such as geology, seismic interpretation, reservoir engineering, production techniques and various other operations.
AI techniques are applied in activities like reservoir characterization, modelling and field surveillance.
Fuzzy logic, expert systems and artificial networks are used to accurately characterize reservoir for optimum production output. Complex logics are required to derive a relationship between critical functions like algorithms defining relationship between seismic attributes, and target lithological properties such as well logs and sand properties.
Production Parameter Optimization – With the oil prices fluctuating new venture in exploration seems to be slowed down, in such a scenario, companies need to optimize the production and manage the decline in production. Decline Curve Analysis (DCA) is one technique used to estimate future production based on the historic data.
However, the well’s decline towards the end of its life, follows a nonlinear pattern, and usually declines quicker at its depletion stage. Production optimization basically deals with the smart management of parameters that will enhance the well’s life such as Pressure, flowrates, and thermal characteristics of injected fluid mixture.
Machine learning algorithms can be used to analyze the sensor data, which is collected in massive volumes to determine the health of the system and to identify the optimum operating environment.
The value of AI and machine learning can be applied in a different statistical model, which can help improving asset management decision. Effective adoption of these learning technique will be dependent on the integration, with data visualization and effective user interface design.
Field Equipment Management – Often the fields and the wells are in remote locations and gathering data on the status of the pumps and well physically, becomes a tedious job. This would sometimes make the data sparse and difficult to access; however, the cost of equipment and the potential it must deliver, needs to be optimized.
With the advance in technology, Oil & Gas IoT sensor data is collected at the server level. This data is enriched with other well information and geological complexities to create a more complete picture with the help of various algorithms of what’s happening in the field, or might happen if certain parameters are to be ignore, for example, situations like equipment failure, its reason for failure, how it arrived at that stage.
If it failed sooner than expected, and can it be avoided in the future? Such techniques help in increasing the life of the equipment with less risk of accidents and lower maintenance cost for best possible results.
Road Ahead
Through the use of deep learning and IoT, new predicting and monitoring technologies for the oil and gas industries have emerged that could completely transform them. Being able to predict what’s coming and see beyond what’s currently seen allows companies to deal with potential problems before they happen, saving them time, money, and bad publicity. A future where we’re surrounded by artificial intelligence incorporating deep learning and IoT is imminent.
Going forward, the impact of ML and AI has already been realized in the industry. Early adopters are taking advantage with a head-start in the competition to protect their assets. Tightening research and development budgets are prompting oil and gas firms and their suppliers to think differently about both how they source new technologies and where they direct scarce resources.
These evolving technology approaches are likely to outlast the current downturn and could lead to real changes in companies’ overall business strategies.
-
News1 month ago
Offer Price, Overview, Investors: Here’s Everything You Need to Know About Asyad Shipping’s Mega IPO
-
OER Magazines1 month ago
OER, February 25
-
Automotive2 months ago
[REVIEW] A Legend Reinvented: The Nissan Patrol Y63 Blends Heritage with Modern Mastery
-
Alamaliktistaad Magazines1 month ago
Al-iktisaad, February 2025
-
Energy1 month ago
Dubai Supreme Council of Energy Unveils 5th Emirates Energy Award 2023–2025 in Muscat
-
News2 months ago
Oil Prices Rise as Investors Assess New US Tariff Threats
-
News2 months ago
Middle East Emerging as a Key Destination for Sports Tourism
-
Banking & Finance1 month ago
Alizz Islamic Bank Partners with Visa to Launch Premium Shari’a-Compliant Card Solutions
You must be logged in to post a comment Login