Leaders Speak
Harnessing the power of data – by Mohamed Nayaz
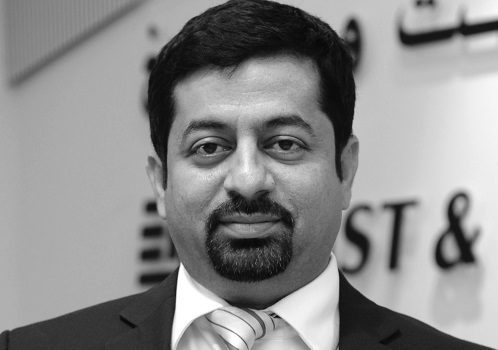
Companies across the world are developing the technical capacity to analyse the vast amount of information they have captured. They are using the insights generated from that data to build stronger customer relationships, reach new customers and improve the bottom line.
According to Gartner, the MENA region’s business intelligence (BI) software revenue is estimated to reach $260mn by the end of 2016. Despite the slow economic growth in some regions, BI is likely to remain one of the fastest growing software markets. This is because organisations consider BI as an important tool in making businesses smarter, more agile and efficient.
Internal audit (IA) uses Big Data and advanced analytics to analyse large amounts of information. BI derived from Big Data helps organisation improve operational effectiveness. BI helps in sharing, summarising and manually exploring data and metrics, whereas advanced analytics helps automate decision making in IA.
Predictive analytics uses Big Data insights and findings for decision making in IA. Today, organisations give importance to analysing why things happen. Organisations are now forecasting what is likely to happen in the future and optimising what should happen based on an increasingly varied set of data sources and types. The addition of mobile, social and collaborative technologies to advanced analytics tools will help a wider set of users in making decisions at the right time.
The IA function must embrace analytics to keep pace with or outpace the business – it must become a natural part of the thought process. This will involve not only adopting new tools and techniques but also changing the mindset. However, the results will be worth the effort. IA’s use of data analytics can help a business improve its processes and deliver even better products and services.
IA AND ANALYTICS
In the context of IA, analytics is the analysis of a large population of data to obtain insights and improve business performance, reduce risk and maximise business value.
Traditionally, an audit focuses on what could go wrong. Auditors attempt to understand the population and build a representative sample that can be extrapolated. The sample might not be a proper representation and the resulting audit might miss critical areas and fail to identify all relevant issues. With the use of analytics, IA can examine an entire population of data using various techniques (e.g., descriptive, predictive, prescriptive) and focus on potential issues.
To stay ahead, IA needs to leverage analytics more. An EY survey conducted in 2013 (respondents included 513 Audit Committee members and Chief Audit Executives, spanning 20 industry sectors, majority of companies had annual global revenue of $250mn and greater), states only 11.7 per cent of respondents said they use analytics in the entire IA process.
While data analytics is not a new concept, few IA functions use analytics throughout the entire audit process.
CHALLENGES
The best way to view the challenge of integrating analytics into the audit is through a model comprising three components – “define”, “produce” and “consume” analytics and supported by “governance” analytics.
The first challenge is the most obvious: how to make sense of the mountains of available data. Auditors need to know what they are looking for. They need to understand the business processes, risks and control objectives to identify the appropriate data for the question at hand and then access the data – often a very difficult task.
The define component requires considerable diligence in planning, because it is vitally important for the auditing team to know what answers it expects its queries to generate. To get the right answers, auditors must ask the right questions.
Asking those questions in the right way requires collaboration with the professionals who will produce the analytics. Finding the right balance of skills and talent needed to produce the analytics is not an easy task. Both the auditors and the analytics professionals must bring the appropriate skill sets to the table. It also requires effective, ongoing communication with IT department. For that to happen, IA must have or develop an understanding of analytics and what it is capable of effecting. Auditors’ knowledge should include a basic understanding of the key systems and methodologies, and the ability to understand the degree of difficulty posed by a particular question. IA also must keep in mind that testing requires significant costs.
The best way to build and maintain the communications channel is by building a strong relationship between the IA and IT teams, with the goal of forming a new, more enduring partnership. Endurance is necessary because persistence is the key to developing robust and effective analytics.
At first, analytics is likely to prove of relatively limited use and cost-efficiency in the short term is often elusive. It takes time to develop effective, targeted queries and several iterations are often necessary. Once the analytics has been produced, it falls to auditors to consume them; to draw conclusions from the information gathered and turn data into insights that can be delivered to the Board and the C-suite.
IA ANALYTICS JOURNEY
Building leading analytics capabilities into the IA function is a journey that will take significant time and effort.There are three stages of analytics, with the mathematical complexity growing with each step:
Descriptive analytics: Reports, visualises and understands what has already happened, either in real time or after the fact.
Predictive analytics: Helps develop an understanding of the underlying relationship between input and outputs to understand why something happened or to predict what will happen in a given scenario or set of scenarios.
Prescriptive analytics: Determines which decision or action will produce the most effective result.
The maturity model provides a useful way to measure an organisation’s progress along the analytics journey. Companies that have no formal approach and no readily available analytics tools are in the “initial” phase. In the next phase, the analytics are repeatable but not formalised; they are not applied consistently or correctly. Companies tend to move smoothly from the first phase to the second. The third phase, “defined”, presents a more significant hurdle.
Companies in the “defined” phase have an established analytics methodology and they enforce their analytics policy. In the IA management champion’s analytics, a key step toward the “managed” phase, the analytics methodology is institutionalised and the management understands the business issues and root causes. The final phase is “optimised”, where the IA function is locked into continuous improvement and continual monitoring.
DEFINE ANALYTICS
In defining analytics, IA should set the objectives for the audit project, taking into account the scope of the audit, the timing, the team and the audit stakeholders. Once IA has set its objectives, it can build analytics to help achieve those objectives. Analytics tends to involve data that goes beyond normal management business information (e.g., amount sold, average price) to lower-level, higher-volume data (e.g., line item detail for purchase orders and invoices). As the volume of available information increases, the appropriate questions change. The best analytics queries are those that have gone through several iterations, each involving refinements based on questions answered and lessons learned through fieldwork.
PRODUCE ANALYTICS
The “produce” component involves the activities most people are thinking of when they think of analytics – specifically, the technical steps involved in the production of the analytical results.Generally, this component can be broken down into three parts: technology, data and analysis.
Technology encompasses the hardware and software tools needed to get the job done. It includes server use and the data storage capacity required.
Data capacity is a key issue because of the sheer amount of data involved: a dive into lower levels of information will bring much more data to the surface. IA is likely to ask questions that bring in 10 times the previous volume of data, as well as the immediate question of how to store and process the data during analysis. There are several other key issues that need to be resolved:
When should the data be extracted?
- How should it be reconciled?
- Does it need to be archived and if so, for how long?
- How long until it can be deleted?
- What audit evidence must be stored?
Analysis involves using the tools to write the query that creates the analytics outputs.
CONSUME ANALYTICS
The consume analytics component involves turning data into insights, which is crucial to the final report. Continuous feedback is the key to making sure that IA is testing the right components. An output might reveal a certain issue, but the follow-up could reveal a legitimate reason or a workaround. In either case, the test must be revisited. The best way to build feedback into the process is through a formal step, where the auditors sit with the analyst who produced the outputs to make the relevant changes or recalibrations.
Part of the feedback loop also involves establishing benchmarks. IA should make sure that recording these previously unknown benchmarks becomes standard operating procedure.
GOVERN ANALYTICS
The final element of an effective analytics process is governance. Governing analytics is about making sure that the team has the skills it needs, that the right technologies are in place and that the right policies and roles have been defined. It involves the management of skills and assets to make sure that the insights are delivered to the Board and the C-suite as promised.
CASE STUDY
Recently, a global industrial company began an IA transformation, driven in part by the establishment of a program designed to integrate analytics into its IA function. The company’s journey had an end goal: own a world-class audit organisation.
The journey began with focused pilot projects that proved the value of analytics to the audit process. The next step was the construction of a three-year roadmap.
Year one focused on optimising audit performance through the creation of an analytics function with a clear strategy and the necessary resources, tools and technology. By the end of the first year, the company had reached the repeatable maturity level.
Year two focused on expanding risk coverage and creating a defined methodology, and process for integrating analytics into the audit delivery. At the end of the second year, the function was at a defined maturity level with pockets of greater maturity.
Year three focused on optimising risk coverage through embedding analytics in the risk assessment process, industrialising the analytics enablers and tools, and further pushing training and awareness throughout the function. In addition, the function instituted a formal analytics governance process.
The results speak for themselves – the company’s IA function now deploys advanced analytics in:
Annual risk assessment
- More than 40 per cent of its audits
- Four to five special projects each year
Full-time analytics resources participate in all stages of the audit life cycle, including fieldwork, and the value of the analytics is tracked for each project. The improved insights, effective audit procedures and efficient delivery of process monitoring analytics have greatly increased the visibility and impact of IA.
KEY BENEFITS:
Audit intelligence: Auditors are using interactive dashboards to facilitate analysis.
Efficiency: Previously built analytics and monitors are produced in half to a third of the initial time.
Value to the business: Process monitoring analytics are used across various locations to provide insights into the process and highlight areas of focus for IA and the business.
CONCLUSION
The year 2012 witnessed a global market growth of business analytics technology and services by 8.8 per cent to touch $95.6bn. IDC forecasts further growth for the market through 2017, at a compound annual growth rate (CAGR) of 10.8 per cen.
IA must integrate BI and analytics into its audit process to keep pace not only with the business, but also with the organisation’s competitors. Analytics is not a “nice to have,” but a “must have,” because IA must build the capability to analyse the vast amount of data generated by the organisation to meet its compliance mandate.
IA provides business insights and acts as a strategic advisor while holding the line on costs or even reducing them. When it comes to Big Data and analytics, the future of IA is now.
(The author is Partner, Advisory Services, MENA Leader for IT Risks and Business Resilience Services, EY)
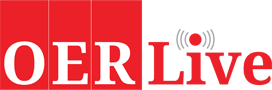
-
Oil & Gas1 month ago
Oman Oil Exports Exceed 135.3m Barrels by End of June 2024
-
Hospitality2 months ago
OMRAN Group Achieves RO31.6M Net Profit, 44.6% Revenue Growth
-
Energy2 months ago
VIDEO: Dr. Firas Al-Abduwani Discusses Green Hydrogen, Potential Regions for Development & Creating a Green Ecosystem
-
Energy2 months ago
Oil Futures Rebounds Over US$1 After 7-Week Low
-
Leaders Speak2 months ago
VIDEO: Discussing The Future of Green Hydrogen in Oman with H.E. Salim Al Aufi
-
Interviews2 months ago
VIDEO: Talking Latest Developments in Aircraft Ground Guidance & More at Oman Airports
-
Automotive2 months ago
Nissan Appoints Al Hashar Automotive as the Authorised Official Distributor in Oman
-
Technology2 months ago
Celebrating Infoline’s Remarkable 20-Year Journey